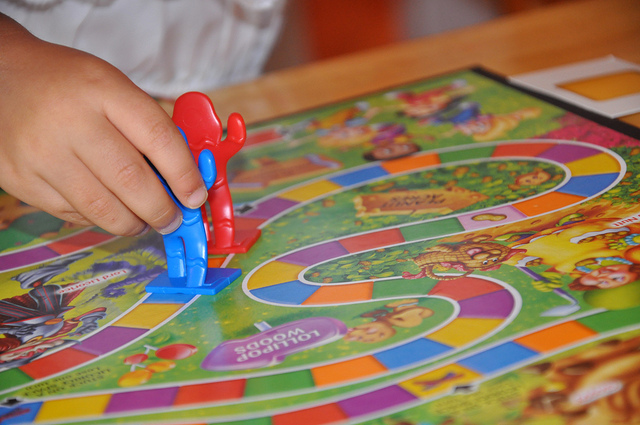
I’ve been spending the last year working on machine learning methods to train an algorithm that could be competitive with professional-level Candyland players. I’m happy to report that as of today, I attained my goal.
For the first pass of my automated Candyland player, I built a very simple rules-based engine. The engine ran efficiently on a Mac Book Pro. The rules-based engine did ok in lab tests with strong amateur players. I knew it couldn’t stand up to the rigor of professional Candyland tournament play, however.
For the second pass of my player, I created a more sophisticated look-ahead engine. Before taking its next card on its turn, it would simulate every possible permutation of the rest of the deck. While this was very processor intensive, by moving it into AWS and distributing it across 100 xLarge instances, it ran near real-time, although at some expense. The results of this player weren’t significantly better than the first engine.
In my third pass, I trained a shallow Convolutional Neural Network with 100,000 Candyland games. The results of this were disappointing. Playing against my look-ahead engine, over 500,000 games, it did no better than winning 50.05% of the time. Adding more layers didn’t improve the score significantly.
The fourth permutation of my Candyland player was a fully recurrent neural network running across a few dozen AWS GPU instances. This player played itself for several weeks improving the weights in its network using a genetic algorithm. The results from this were fascinating. It was able to win against itself 100% of the time.
This morning, April 1st, 2018, I played three games against the RNN player. While I am not a professional level Candyland player, I am rated 1200 on the amateur circuit, and it was my favorite game in 1st grade. This last version of my player beat me all three times! I think it is finally ready.
I’m going to be setting up some exhibition games with my Candyland player as soon as I can track down someone on the professional Candyland circuit. Not only will this gain some positive publicity for machine learning, but I’m hoping that it will help me recoup the $350,000 in AWS bills that I racked up building, testing, and training my players.